Log Odds and Odds Calculator In statistics, odds, log odds and expected proportion are three different ways of expressing probabilities, which are related to each other You can find out the value of one of these by knowing the value of any two Here is the free online Log odds and odds calculator for calculation of log odds, odds or expectedThe coefficient returned by a logistic regression in r is a logit, or the log of the odds To convert logits to odds ratio, you can exponentiate it, as you've done above To convert logits to probabilities, you can use the function exp (logit)/ (1exp (logit)) However, there are some things to note about this procedureIn 1944, Joseph Berkson used log of odds and called this function logit, abbreviation for "logistic unit" following the analogy for probit Log odds was used extensively by Charles Sanders Peirce (late 19th century) G A Barnard in 1949 coined the commonly used term logodds;
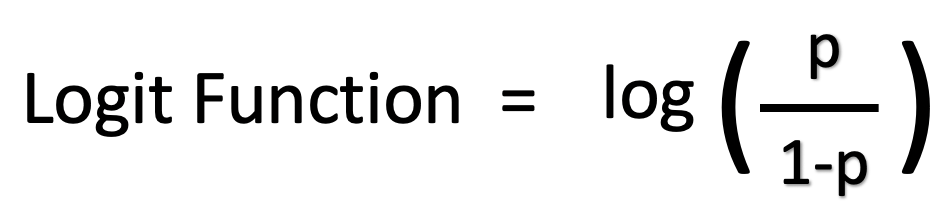
What And Why Of Log Odds What Are Log Odds And Why Are They By Piyush Agarwal Towards Data Science
Convert log odds to odds ratio
Convert log odds to odds ratio- The difference between cats and dogs is 3 percentage points or 3 49 ≈ 612 % increase in probability for dogs over cats Meanwhile the odds ratio is 052 1 − 052 049 1 − 049 ≈ 1128 However I am finding a lot of social science literature intpreting O R − 1 as equivalent to increase in probability Example Crude Odds Ratio – the odds ratio calculated using just the odds of an outcome in the intervention arm divided by the odds of an outcome in the control arm Adjusted Odds Ratio – is the crude odds ratio produced by a regression model which has been modified (adjusted) to take into account other data in the model that could be for instance a prognostic baseline variable




Log Odds Ratio Plot Of Tnt Vs Diagnoses Download Scientific Diagram
Risk ratios, odds ratios, and hazard ratios are three common, but often misused, statistical measures in clinical research In this paper, the authors dissect what each of these terms define, and provide examples from the medical literature to illustrate each of these statistical measures Finally, the correct and incorrect methods to use these measures are summarizedFor the constant, B = logodds that the 0/1 coded outcome variable is a 1 when all explanatory variables = 0 (or reference category for categorical variables)Odds ratio is the likelihood that an event will occur in relation to the likelihood that an event will not occur, 1 event for and 5 events against In Gambling, the "odds" are a ratio of the likelihood of a certain outcome, related to the other outcomes I had to look this up, because I forgot this part of finite math, from 25 years ago
LOG ODDS RATIO Name LOG ODDS RATIO (LET) Type Let Subcommand Purpose Compute the bias corrected log odds ratio between two binary variables Description Given two variables where each variable has exactly two possible outcomes (typically defined as success and failure), we define the odds ratio asFor all 've' values of x, log x can vary between ∞ to ∞ So far we have understood odds Let's describe Odds ratio, which as the name suggests, is the ratio of oddsConsidering the example above, Odds ratio, represents which group (male/female) has better odds of success, and it's given by calculating the ratio of odds for each groupIn video two we review / introduce the concepts of basic probability, odds, and the odds ratio and then apply them to a quick logistic regression example Un
216 Odds ratios and logistic regression ln(OR)=ln(356) = −1032SEln(OR)= 1 26 1 318 1 134 1 584 = 95%CI for the ln(OR)=−1032±196×2253 = (−1474,−590)Taking the antilog, we get the 95% confidence interval for the odds ratio 95%CI for OR=(e−1474,e−590)=(229,554) As the investigation expands to include other covariates, three popular approachesEnglishwise, they are correct it is the odds and the odds are based on a ratio calculation It is not , however, the odds ratio that is talked about when results are reported The odds ratio when results are reported refers to the ratio of two odds or, if you prefer, the ratio of two odds ratios That is, let us writeOdds ratios and logistic regression When a logistic regression is calculated, the regression coefficient (b1) is the estimated increase in the log odds of the outcome per unit increase in the value of the exposure In other words, the exponential function of the regression coefficient (e b1) is the odds ratio associated with a oneunit increase in the exposure
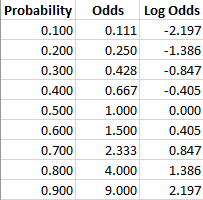



Log Odds Definition And Worked Statistics Problems



Odds Ratio For A Simple Distribution Jmp User Community
The coefficient for female is the log of odds ratio between the female group and male group log(1809) = 593 So we can get the odds ratio by exponentiating the coefficient for female Most statistical packages display both the raw regression coefficients and the exponentiated coefficients for logistic regression modelsLabs(title ="probability versus odds") 000 025 050 075 100 0 50 100 150 odds p probability versus odds Finally, this is the plot that I think you'llfind mostThe odds ratio is simply the ratio between the following two ratios The ratio between standard treatment and the new drug for those who died, and the ratio between standard treatment and the new drug for those who survived From the data in the table 1, it is calculated as follows OR = (a/b)/ (c/d) = (152/17)/
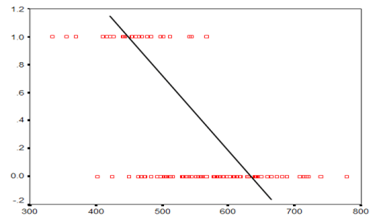



Logistic Regression Understanding Odds And Log Odds By Shruti Wagh Wicds Medium



Plos One Elucidating The Foundations Of Statistical Inference With 2 X 2 Tables
Log odds are an alternate way of expressing probabilities, which simplifies the process of updating them with new evidence Unfortunately, it is difficult to convert between probability and log odds The log odds is the log of the odds Thus, the log odds of A are l o g i t ( P ( A)) = l o gIn our example above, p wine and p no_wine were 0009 and 0012 respectively, so the odds ratio was a good approximation of the relative risk OR = 0752 and RR = 075 If the risks were 08 and 09, the odds ratio and relative risk will be 2 very different numbers OR = 044 and RR = 0 Relative risk vs Odds ratio Summary Our theoretical Odds Ratio is 0319 with a CI(0, 041), which is close to the true Odds ratio, 03This indicates if the undergraduate students are from the school in prestige 3 or 4, the chances of them getting in graduate school is 38% that of the students from prestige 1 or 2 undergraduate schools




Odds Ratios Need To Be Graphed On Log Scales Andrew Wheeler




File Odds Ratio Map Svg Wikimedia Commons
Figure 1 log x vs x;The odds ratio An odds ratio (OR) is a measure of association between an exposure and an outcome In a casecontrol study you can compare the odds that those with a disease will have been exposed to the risk factor, with the odds that those who don't have the disease or condition will have been exposed321 Log odds ratio First, the log odds of word w w in document i i is defined as logOi w = log fi w 1−fi w log O w i = log f w i 1 − f w i Logging the odds ratio provides a measure that is symmetric when comparing the usage of word w w across different documents Log odds ratio of word w w between document i i and j j is
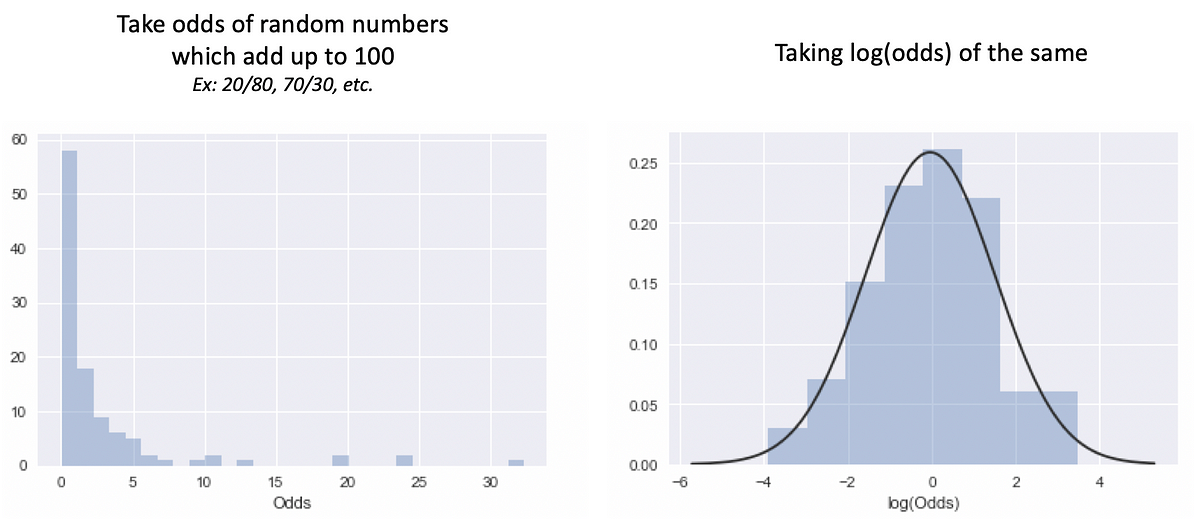



What And Why Of Log Odds What Are Log Odds And Why Are They By Piyush Agarwal Towards Data Science



1
The risk or odds ratio is the risk or odds in the exposed group divided by the risk or odds in the control group A risk or odds ratio = 1 indicates no difference between the groups A risk or odds ratio > 1 indicates a heightened probability of the outcome in the treatment group The two metrics track each other, but are not equal Logs are not very intuitive, so that's why we use the Odds Ratio instead So that Odd Ratio of 97 is still the effect of X going up one unit For each one unit increase in the predictor X, the odds of a success occurring is only 97 times as bigOdds are a ratio of an event occurring to an event not occurring Now let us define probability in this case and the difference between odds and probability will be clear The event occurring/ (the event occurring the event not occurring) With the help of the example above, the ratio would then equate to = 3/8 This is the probability of winning




5 Multiple Logistic Regression 1
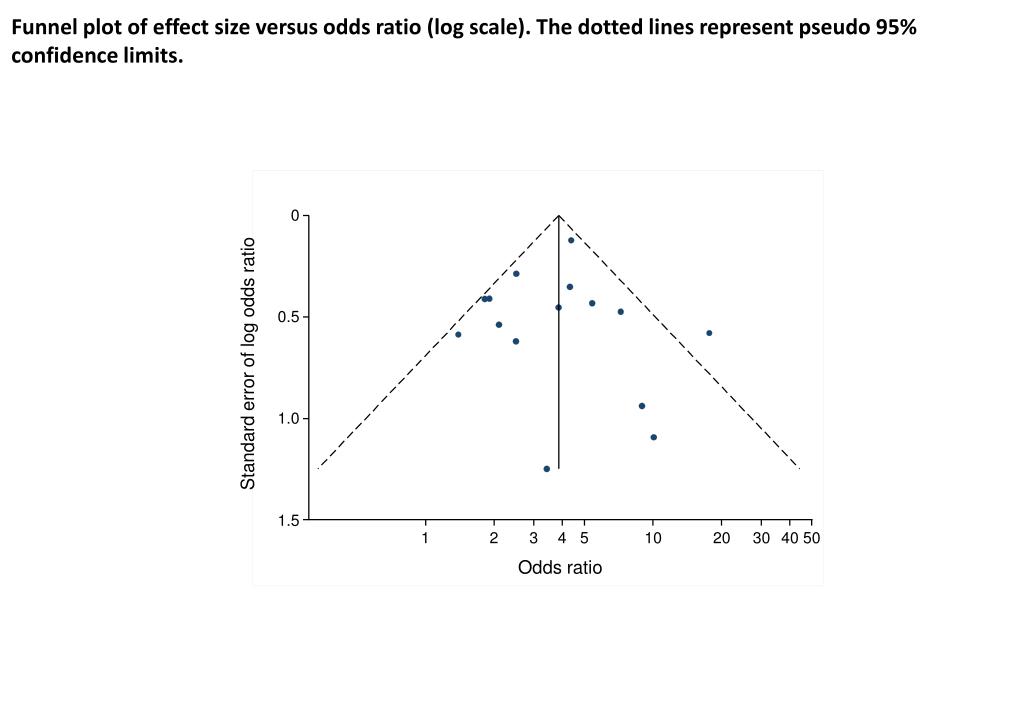



Ppt 0 5 Powerpoint Presentation Free Download Id
When x3 increases from 1 to 2, the logodds increases r2r1 When x3 increases from 2 to 3, the logodds increases r3r2 Which corresponds to the estimate for x3 above The odds ratio, is the exponentiation of the difference of the logodds > exp(r2r1) Or, the ratio of the exponentiation of each of the odds The odds of an event represent the ratio of the (probability that the event will occur) / (probability that the event will not occur) This could be expressed as follows Odds of event = Y / (1Y) So, in this example, if the probability of the event occurring = 080, then the odds are 080 / (1080) = 080/0 = 4 (ie, 4 to 1) If a raceAn odds ratio (OR) is a statistic that quantifies the strength of the association between two events, A and B The odds ratio is defined as the ratio of the odds of A in the presence of B and the odds of A in the absence of B, or equivalently (due to symmetry), the ratio of the odds of B in the presence of A and the odds of B in the absence of A
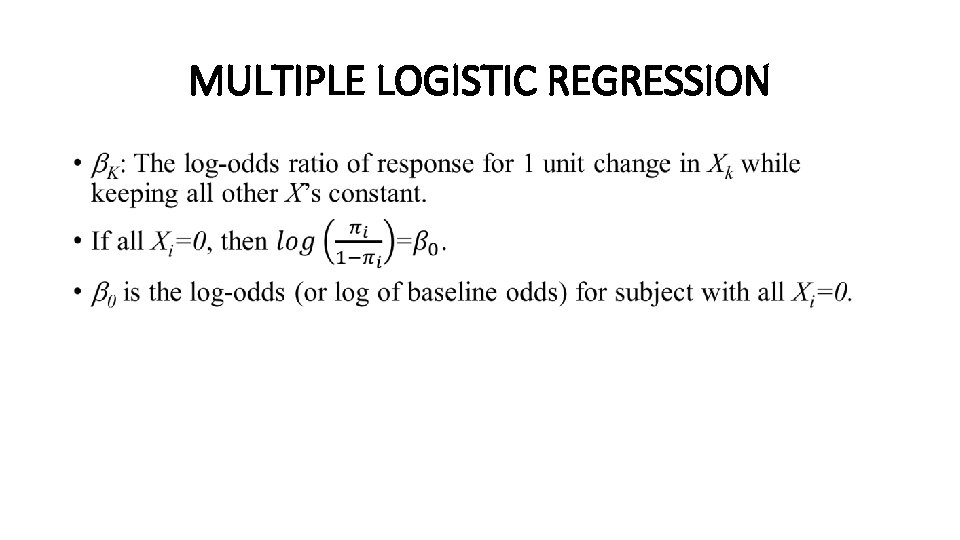



Introduction To Categorical Data Analysis Odds Ratio Measure
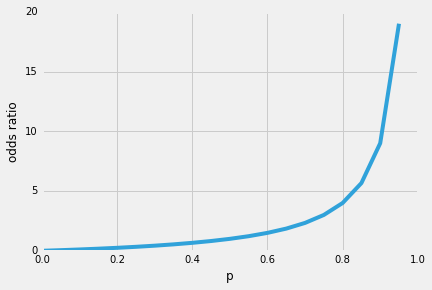



Logistic Regression Why Sigmoid Function
Marginal Effects vs Odds Ratios Models of binary dependent variables often are estimated using logistic regression or probit models, but the estimated coefficients (or exponentiated coefficients expressed as odds ratios) are often difficult to interpret from a practical standpoint Empirical economic research often reports 'marginal effects The odds ratio (OR) is the ratio of odds of an event in one group versus the odds of the event in the other group An RR (or OR) of 10 indicates that there is no difference in risk (or odds) between the groups being comparedOdds, Log odds and exponents This asymmetry problem disappears if we take the 'log' of the odds ratio (OR) 'Log' doesn't refer to some sort of statistical deforestation rather a mathematical transformation of the odds which will help in creating a regression model
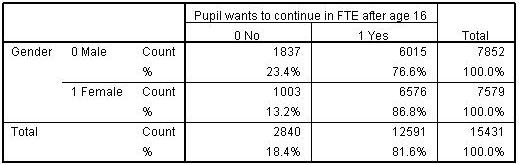



4 2 An Introduction To Odds Odds Ratios And Exponents




Top Pdf Log Odds Ratio 1library
The odds ratio is the ratio of two odds ODDS RATIO Odds Ratio = Odds of Event A / Odds of Event B For example, we could calculate the odds ratio between picking a red ball and a green ball The probability of picking a red ball is 4/5 = 08 The odds of picking a red ball are (08) / 1(08) = 08 / 02 = 4 The odds ratio for picking a red The log odds are modeled as a linear combinations of the predictors and regression coefficients β0 β1xi β 0 β 1 x i The complete model looks like this This equation shows, that the linear combination models the Logit and model coefficients β β descibe the influence of the predictors X X on the logit, eg a one unit increase in xi xThe Log Odds Chart is central to the Logistic Regression ModelIt's with its aid that the slope and intercept of the Logistic Regression Model are calculated And the algorithm is quite simple As mentioned previously, it's quantilebased and, in fact, just a few additional calculations are required to evaluate the regression parameters
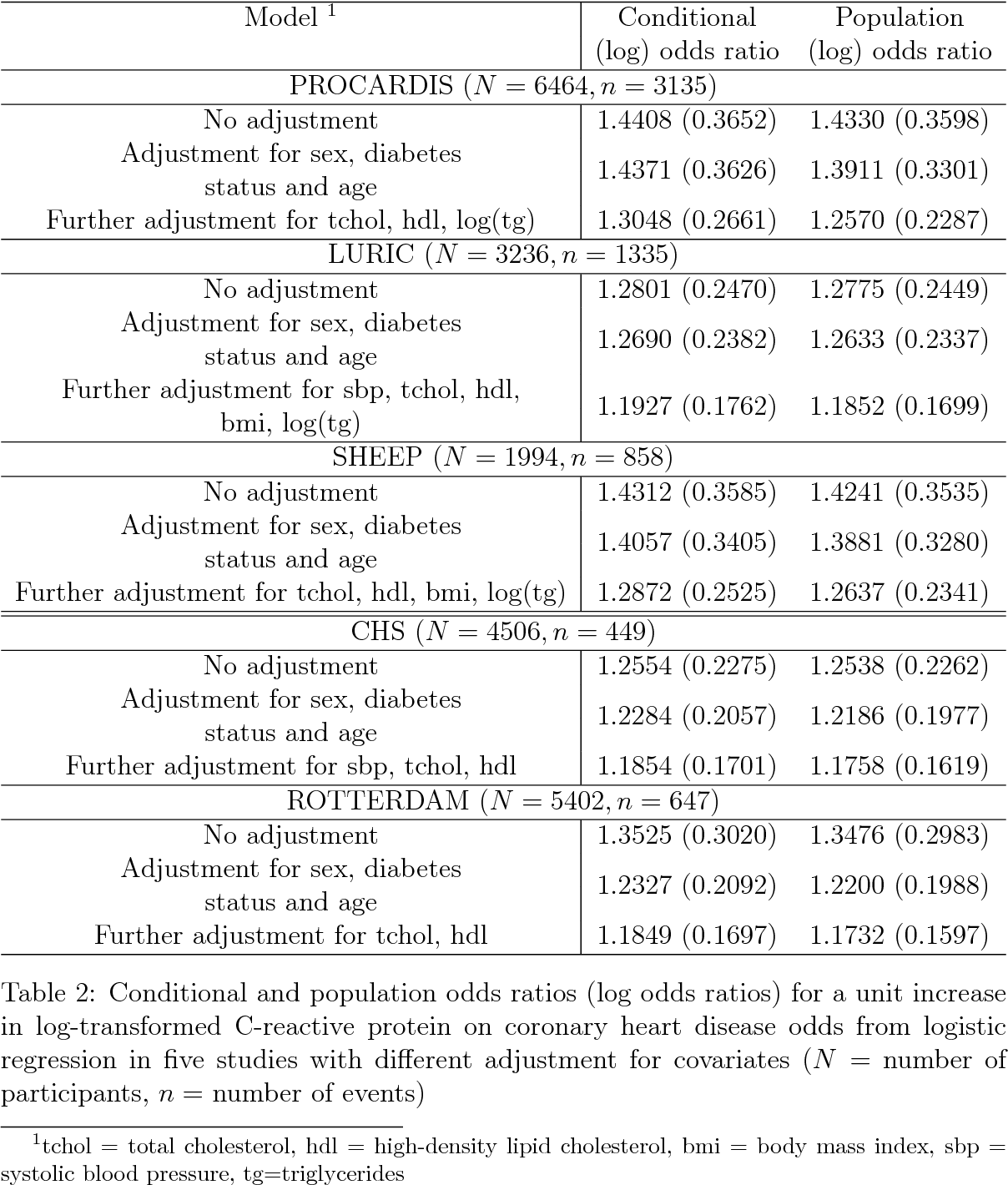



Table 2 From Identifying The Odds Ratio Estimated By A Two Stage Instrumental Variable Analysis With A Logistic Regression Model Semantic Scholar
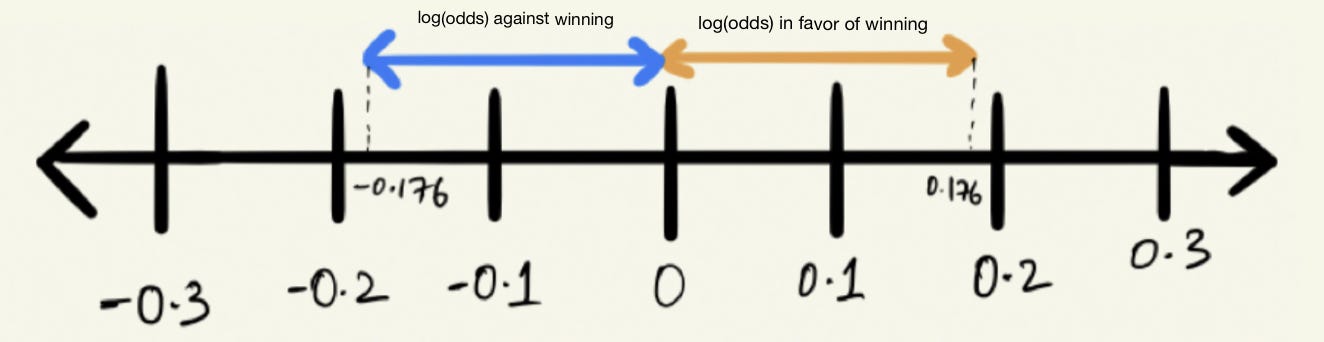



What And Why Of Log Odds What Are Log Odds And Why Are They By Piyush Agarwal Towards Data Science
Odds ratio of having disease for sector 2 vs sector 1 ( 35/44)/(22/95) = 345 (The odds of having disease living in sector 2 is more than 3 times of the odds for living in sector 1) Estimate and compare proportions of persons who contracted the disease by sector, using first 50% of the data Figure2 Odds as a fraction Odds should NOT be confused with Probabilities Odds are the ratio of something happening to something not happeningIn our scenario above, the odds are 4 to 6 Whereas, Probability is the ratio of something happening to everything that could happenSo in the case of our chess example, probability is 4 to 10 (as there were 10 gamesWe can easily transform log odds into odds ratios by exponentiating the coefficients (b coeffcient= 0477)




Log Odds Ratio




Odds Ratios Diagnostic Group Vs Asymptomatic Controls Nominal Download Table
The basic difference is that the odds ratio is a ratio of two odds (yep, it's that obvious) whereas the relative risk is a ratio of two probabilities (The relative risk is also called the risk ratio) Let's look at an example Suppose you have a school that wants to test out a new tutoring programThe logodds of an event is the logit of the probability of the event However,log odds do not provide an intuitively meaningful scale to interpret the change in the outcome variable Taking the exponent of the log odds allows interpretation of the coefficients in terms of Odds Ratios (OR) which are substantive to interpret;
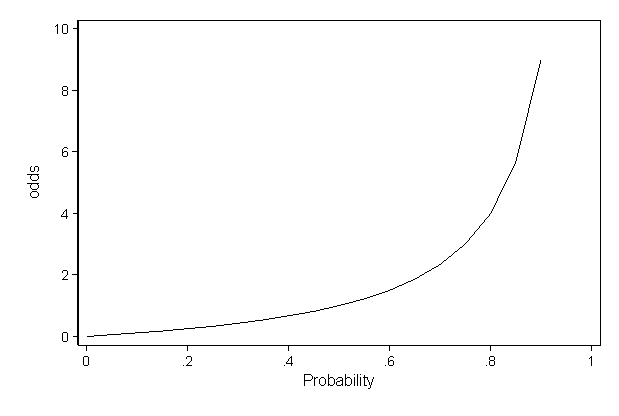



Faq How Do I Interpret Odds Ratios In Logistic Regression
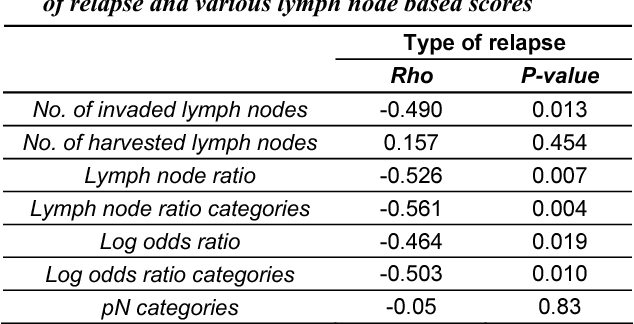



Pdf Lymph Node Status Assessed Through The Log Odds Ratio A Better Tool In The Prognosis Of Colorectal Cancer Relapse Semantic Scholar
The odds ratio is always positive, although the estimated log odds can be positive or negative (log odds of −02 equals odds ratio of 0 = exp(−02)) The odds ratio for a risk factor contributing to a clinical outcome can be interpreted as whether someone with the risk factor is more or less likely than someone without that risk factor to experience the outcome of interest Risk Ratio vs Odds Ratio Whereas RR can be interpreted in a straightforward way, OR can not A RR of 3 means the risk of an outcome is increased threefold A RR of 05 means the risk is cut in half But an OR of 3 doesn't mean the risk is threefold;If you express these as log odds rather than odds ratios, then log odds follow a z distribution, and the Wald test for a single logodds score (ie to see if it's significantly different than




Histogram Showing The Distribution Of The Log Odds Ratios Ors Of The Download Scientific Diagram




Odds Ratio Visualization Tidyverse Rstudio Community
e x p ( β) = odds ratio = p 1 1 − p 1 p 2 1 − p 2 I guess what's not coming across is how β, not being a ratio of odds, converts to the odds ratio metric, when taken out of logarithmic space To provide a bit more, if this is the logistic regression equation for the constant l o g ( p 1 − p) = β β 1 ∗ 0 β 2 ∗ 0 β 3 ∗ 0
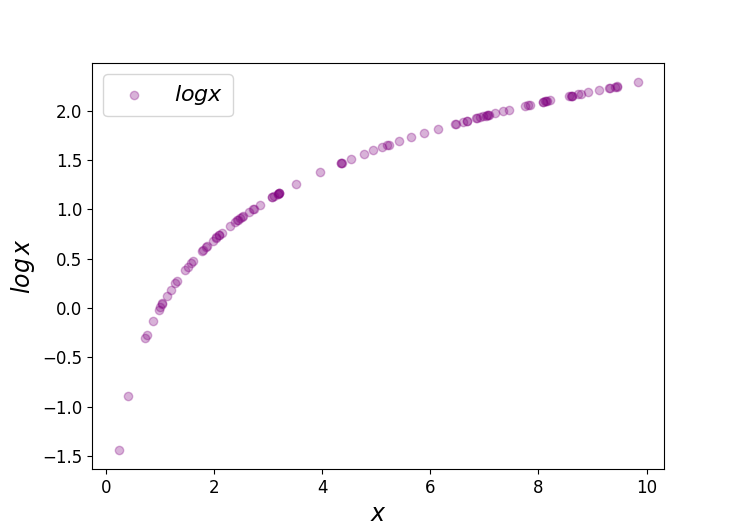



Logit Of Logistic Regression Understanding The Fundamentals By Saptashwa Bhattacharyya Towards Data Science
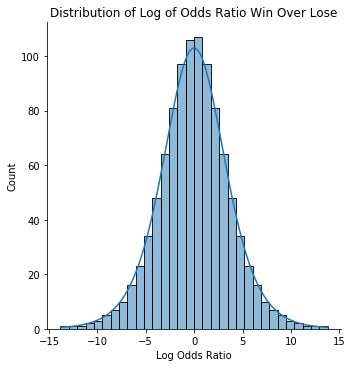



What Are The Odds That You Know About The Odds Analytics Vidhya




Log Odds Ratio Analytics Function Series By Analyttica Datalab Medium



Odds



Boxplots Of The Estimated Log Odds Ratios In 10 000 Simulated Download Scientific Diagram




Statistics Sunday Everyone Loves A Log Odds Ratio Deeply Trivial
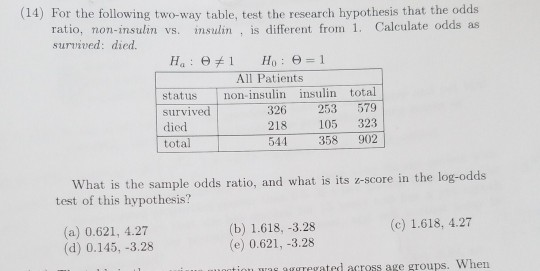



Solved 14 For The Following Two Way Table Test The Chegg Com




Odds Ratios Are Far From Portable A Call To Use Realistic Models For Effect Variation In Meta Analysis Journal Of Clinical Epidemiology




Significant P Value But Odds Ratio Confidence Interval Crosses 1 In Logistic Regression Using Restricted Cubic Splines Cross Validated
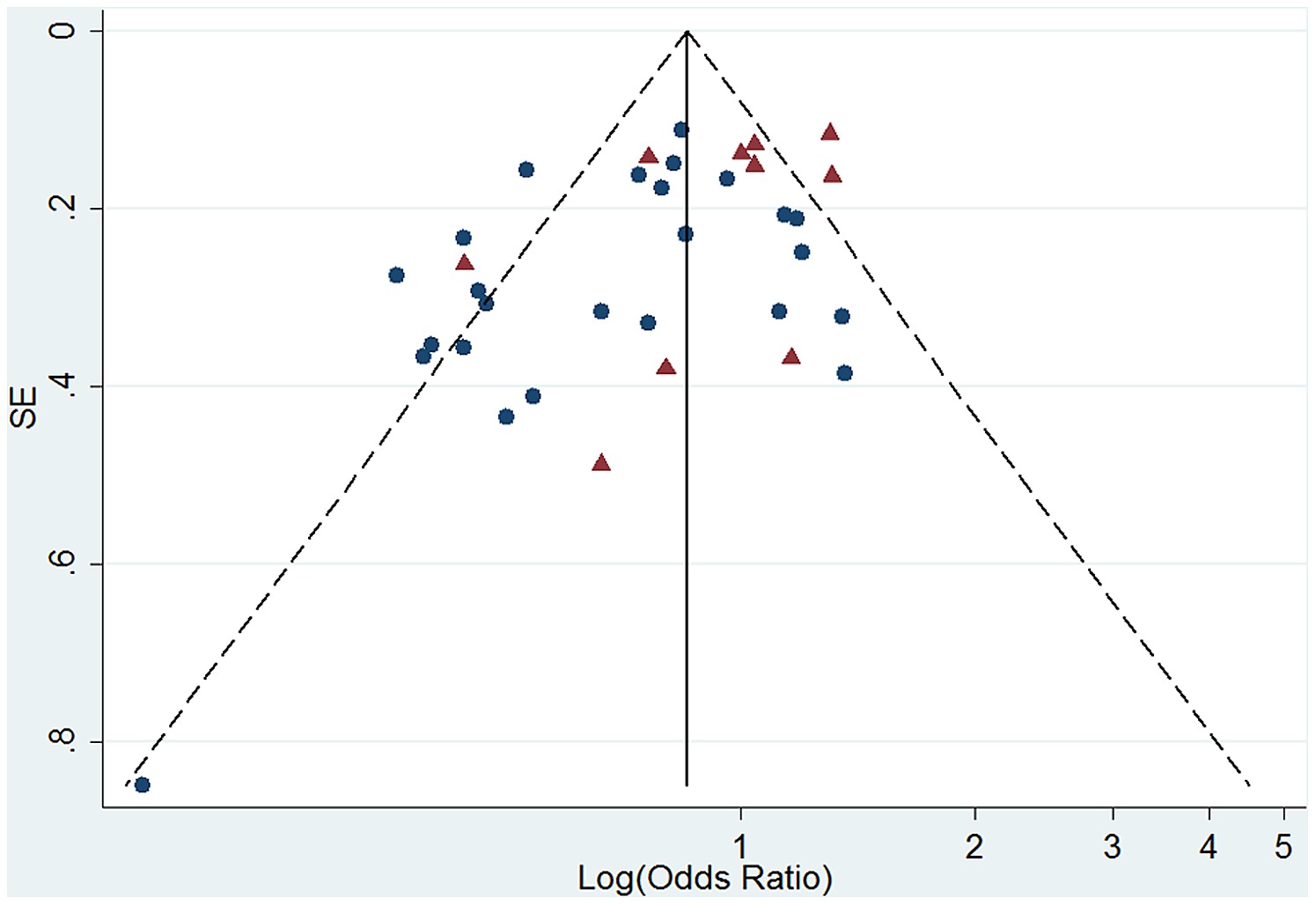



Funnel Plot Of Log Odds Ratio For High Versus Low Soy Isoflavone Intake And Standard Error Se Of Log Odds Ratio Among Premenopausal Women
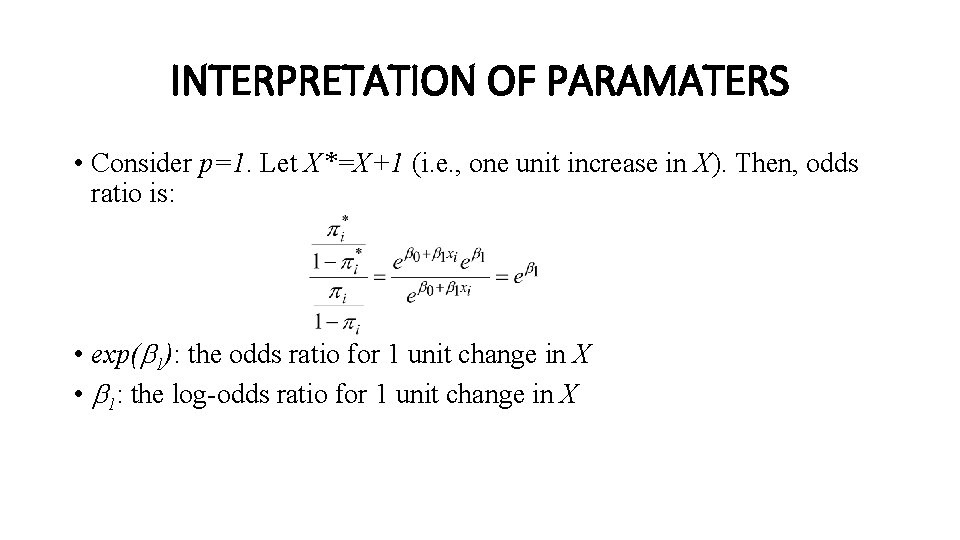



Introduction To Categorical Data Analysis Odds Ratio Measure
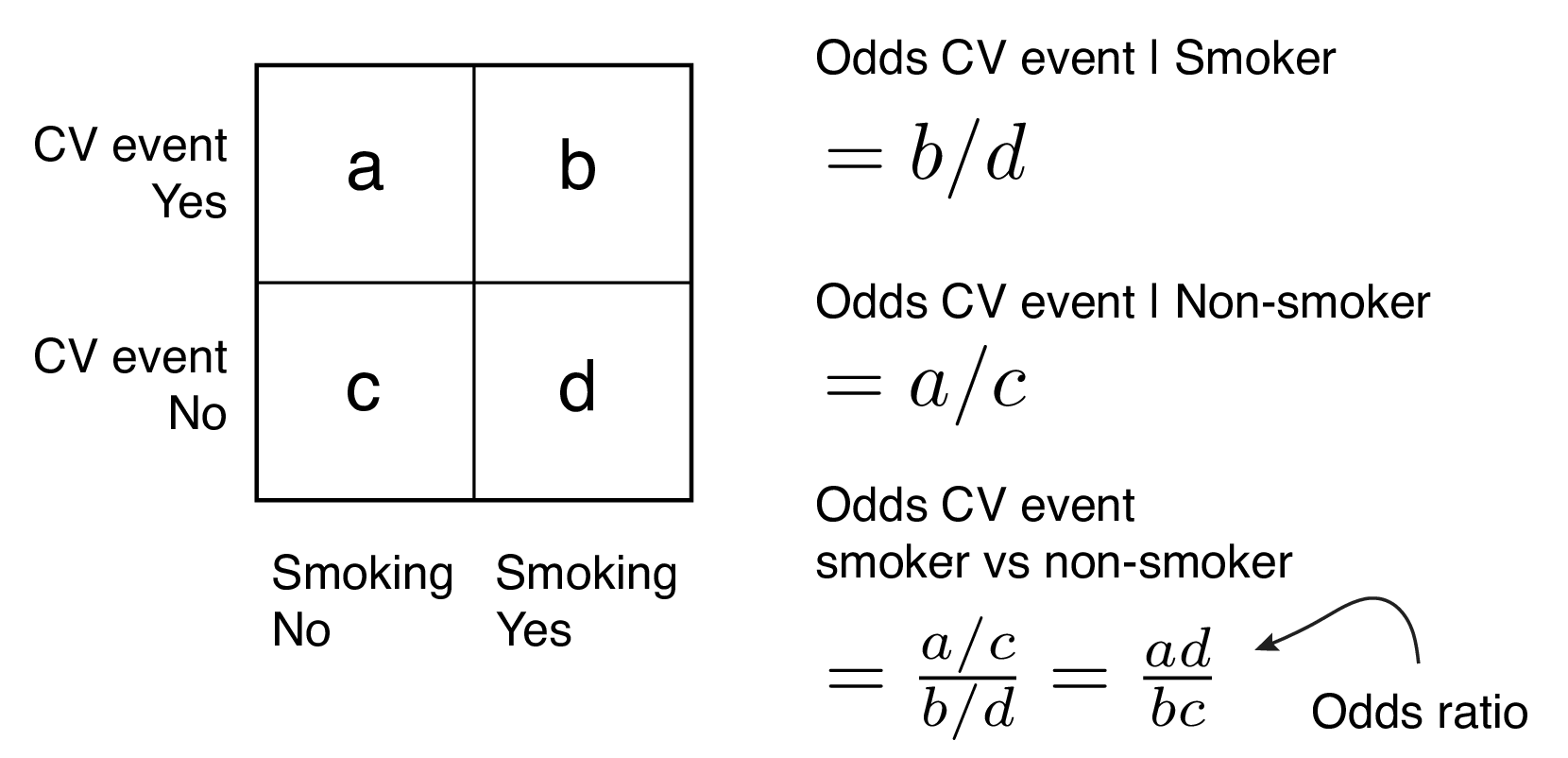



9 2 Binary Logistic Regression R For Health Data Science




Log Odds And The Interpretation Of Logit Models Norton 18 Health Services Research Wiley Online Library



Epos Trade




Probability Curve For The Odds Ratios Of A Logit Model Stack Overflow




Strategies For Graphing Distributions Of Log Odds Estimates And The Corresponding Odds Ratios Modeling The Stan Forums



1




Odds Ratio The Odds Ratio Is Used To Find The By Analyttica Datalab Medium
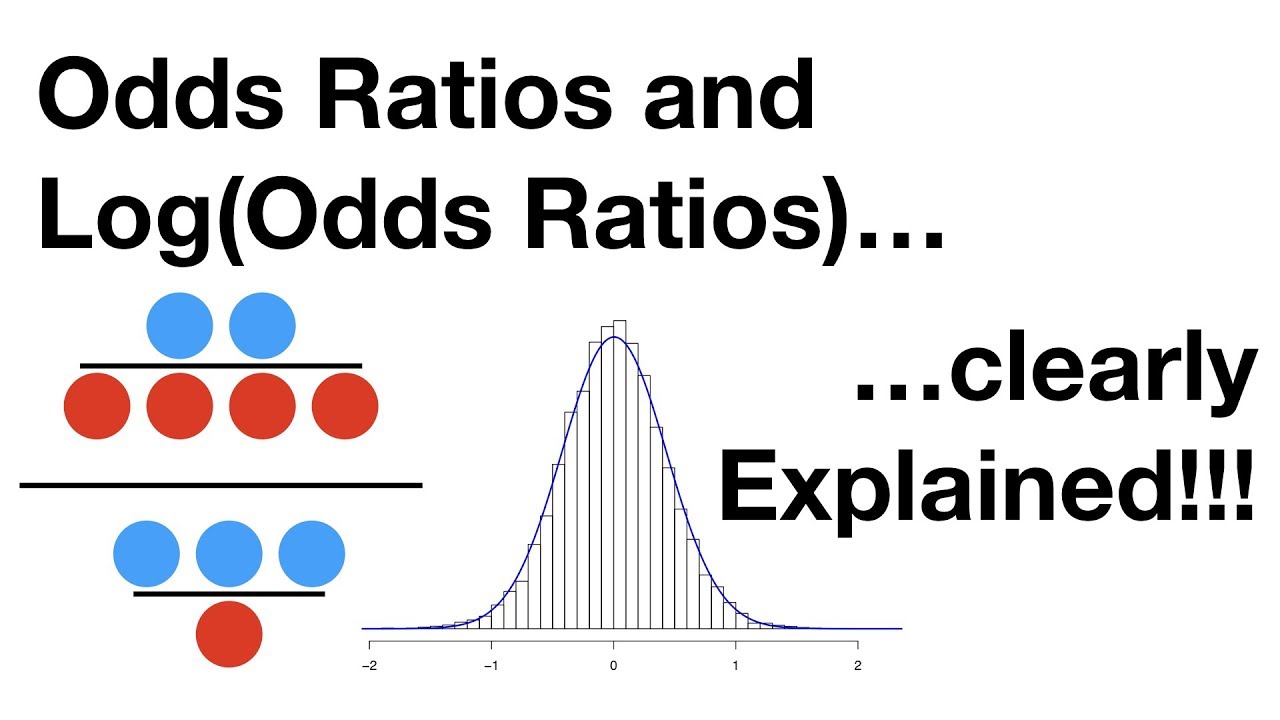



Odds Ratios And Log Odds Ratios Clearly Explained Youtube
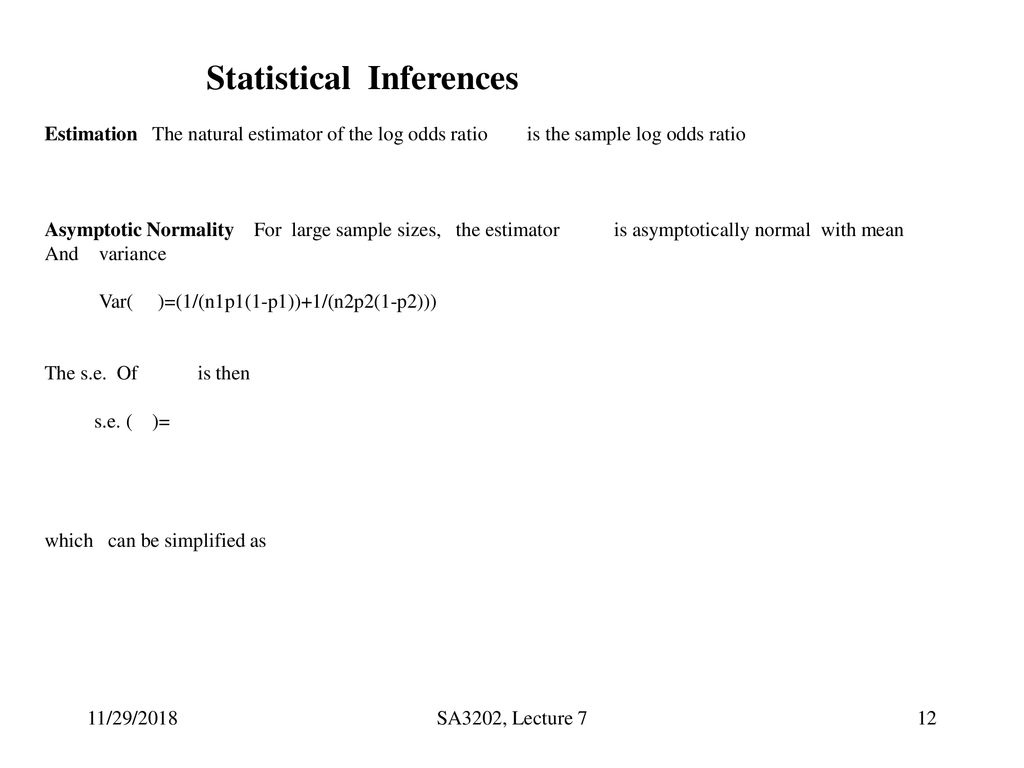



Lecture 7 The Odds Log Odds Ratios Ppt Download
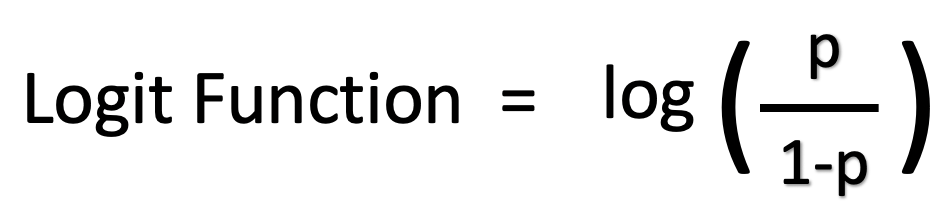



What And Why Of Log Odds What Are Log Odds And Why Are They By Piyush Agarwal Towards Data Science




R Calculate And Interpret Odds Ratio In Logistic Regression Stack Overflow
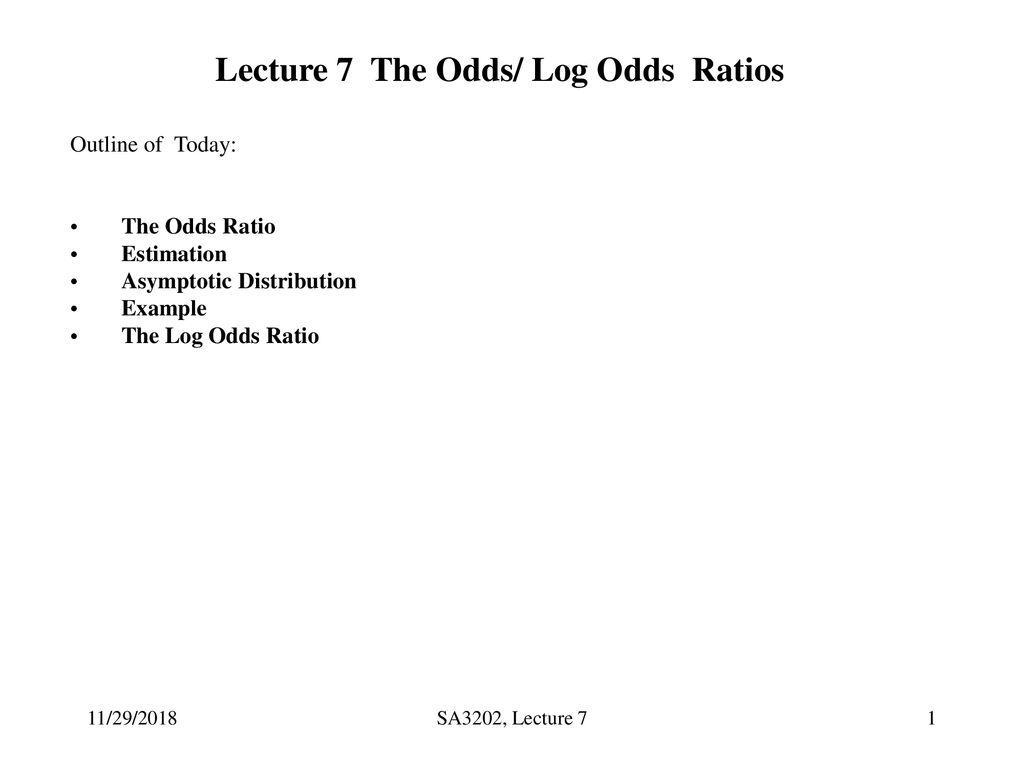



Lecture 7 The Odds Log Odds Ratios Ppt Download




What Is An Odds Ratio And How Do I Interpret It Critical Appraisal




Statistical Analysis Sc504 Hs927 Spring Term Ppt Video Online Download



1




How To Show Odds Ratio Instead Of Log Odds In Bplot From Lrm Model Rms Package Stack Overflow



Forest Plot Of The Log Of The Odds Ratio And Its 95 Confidence Download Scientific Diagram



Plos One Statistical Learning For Turboshaft Helicopter Accidents Using Logistic Regression
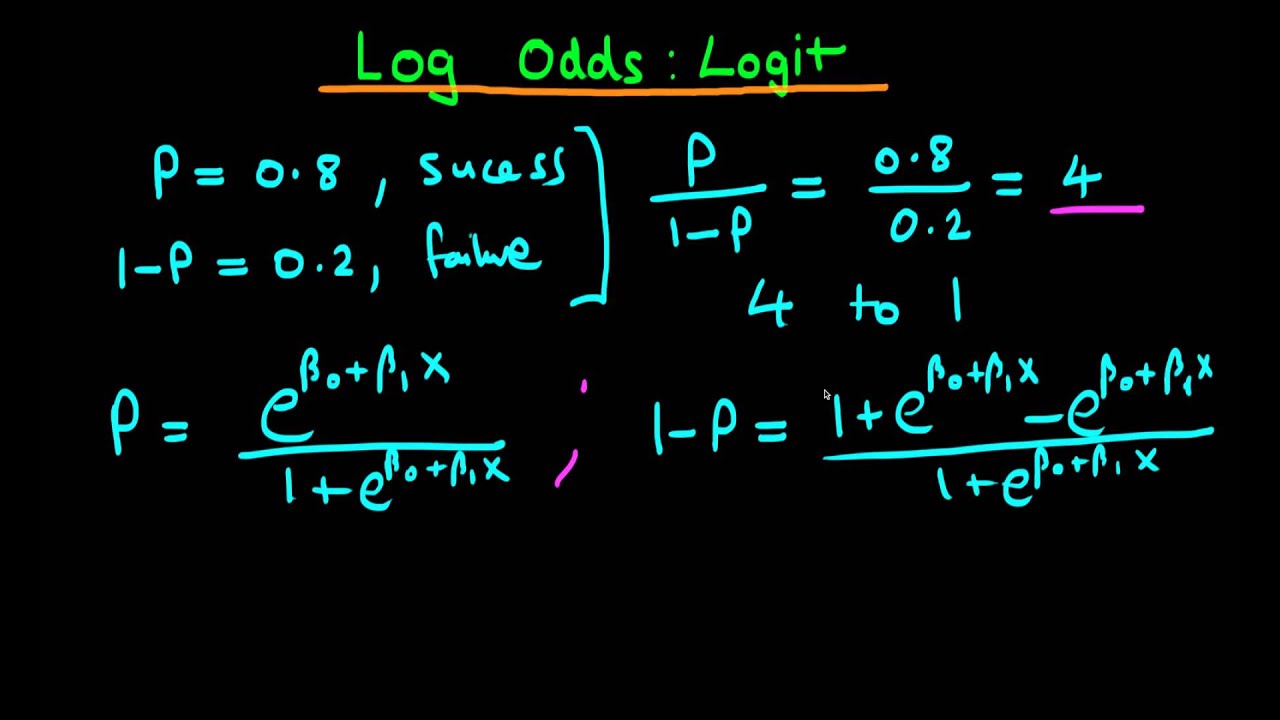



Log Odds Interpretation Of Logistic Regression Youtube




Funnel Plots Comparing Log Odds Ratio Or Versus The Standard Error Of Download Scientific Diagram



2
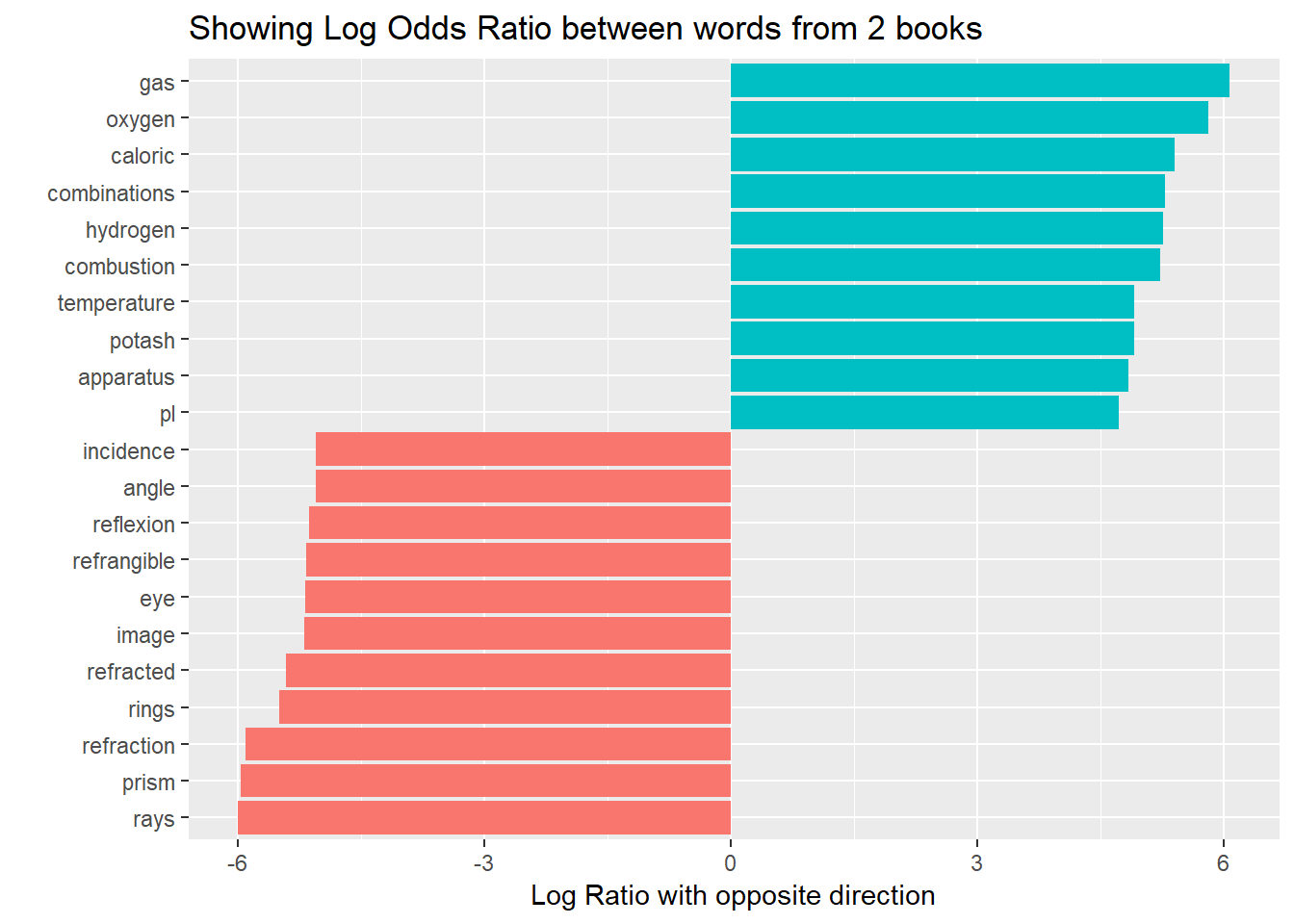



Log Odds Ratio Vs Tf Idf Vs Weighted Log Odds
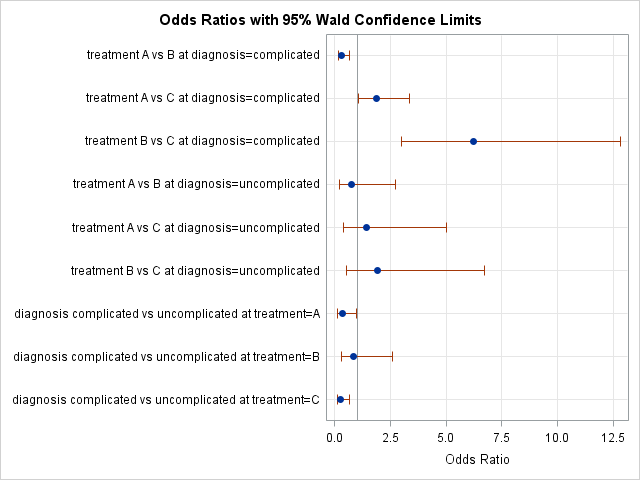



Odds Ratio Plots With A Logarithmic Scale In Sas The Do Loop



How To Get Odds Ratio In Ordinal Logistic Regression Jmp User Community



Wald Vs Likelihood Ratio Test The Stats Geek
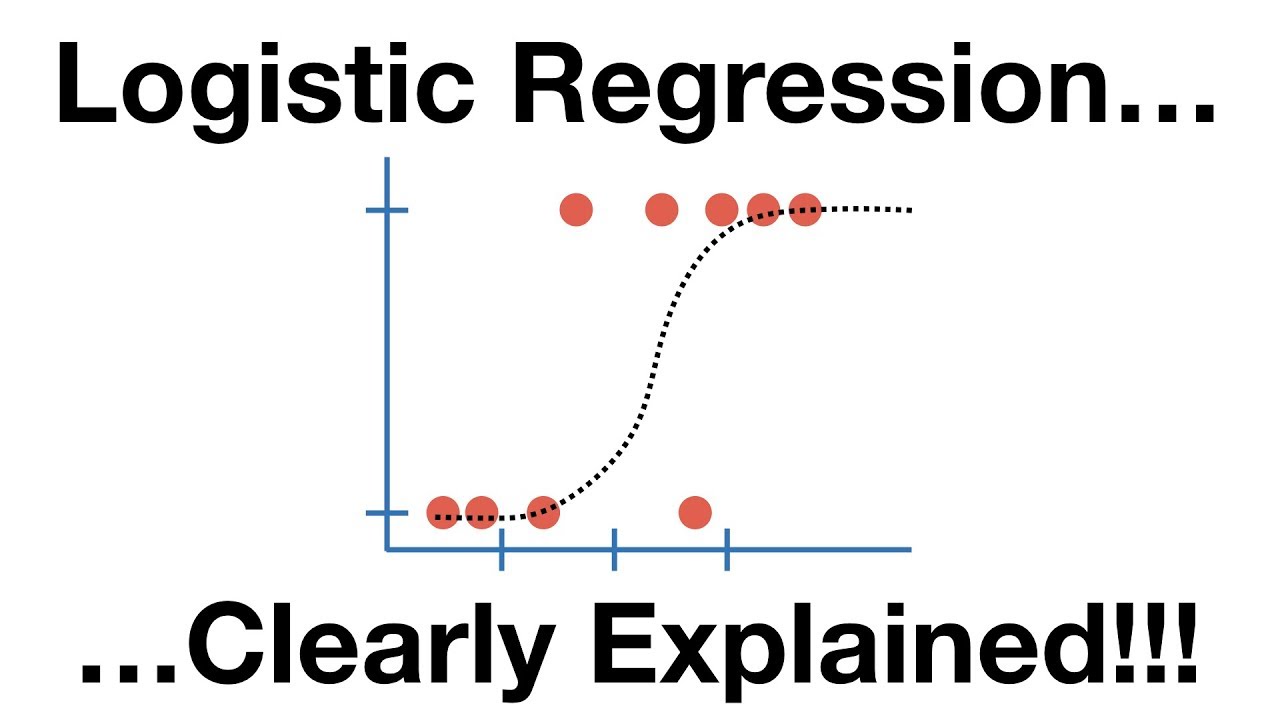



Odds Ratios And Log Odds Ratios Clearly Explained Youtube
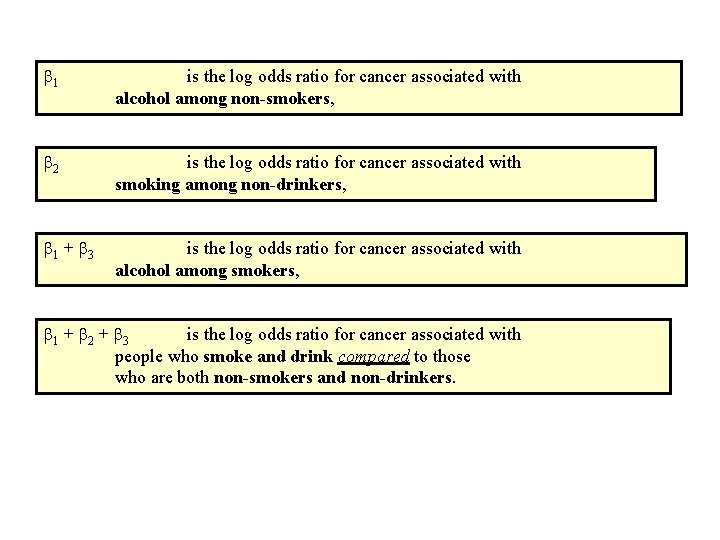



Iv Multiple Logistic Regression V Extend Simple Logistic




Log Odds And The Interpretation Of Logit Models Norton 18 Health Services Research Wiley Online Library




Frequency Vs Log Odds Ratio For Each Word Positive Odds Ratios Download Scientific Diagram
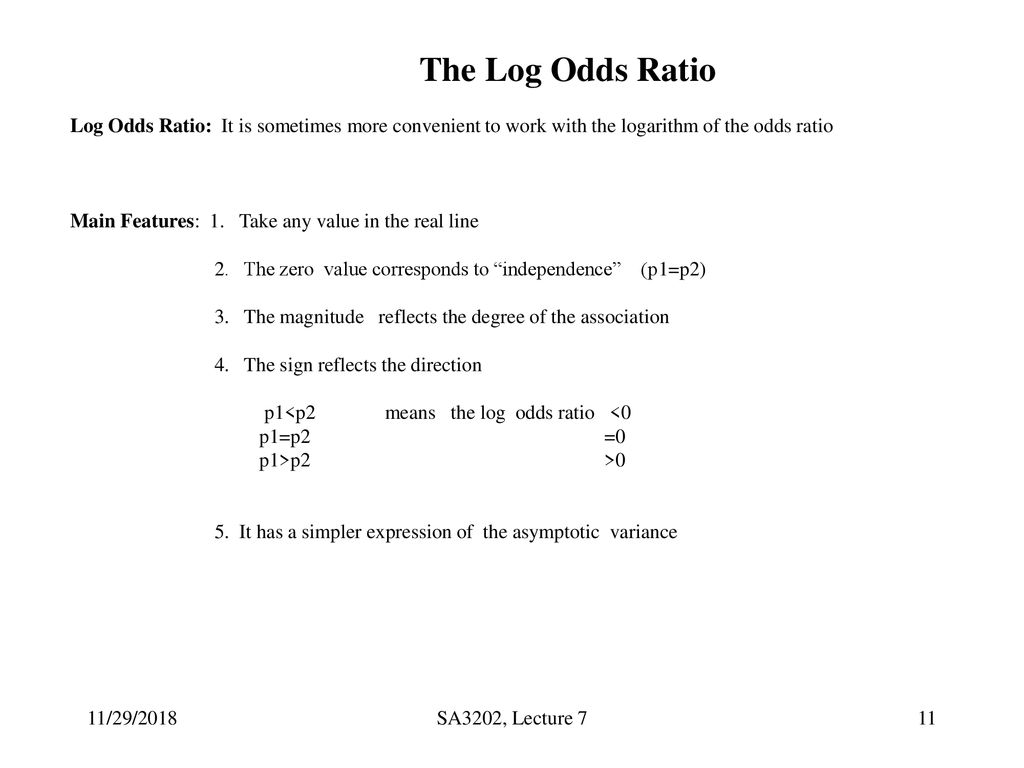



Lecture 7 The Odds Log Odds Ratios Ppt Download




The Gam Smoothing Plot Shows The Log Odds Ratio To Predict Cure Of Download Scientific Diagram




Log Odds Ratios From Snp Drug Resistance Associations Are A Potential Download Scientific Diagram
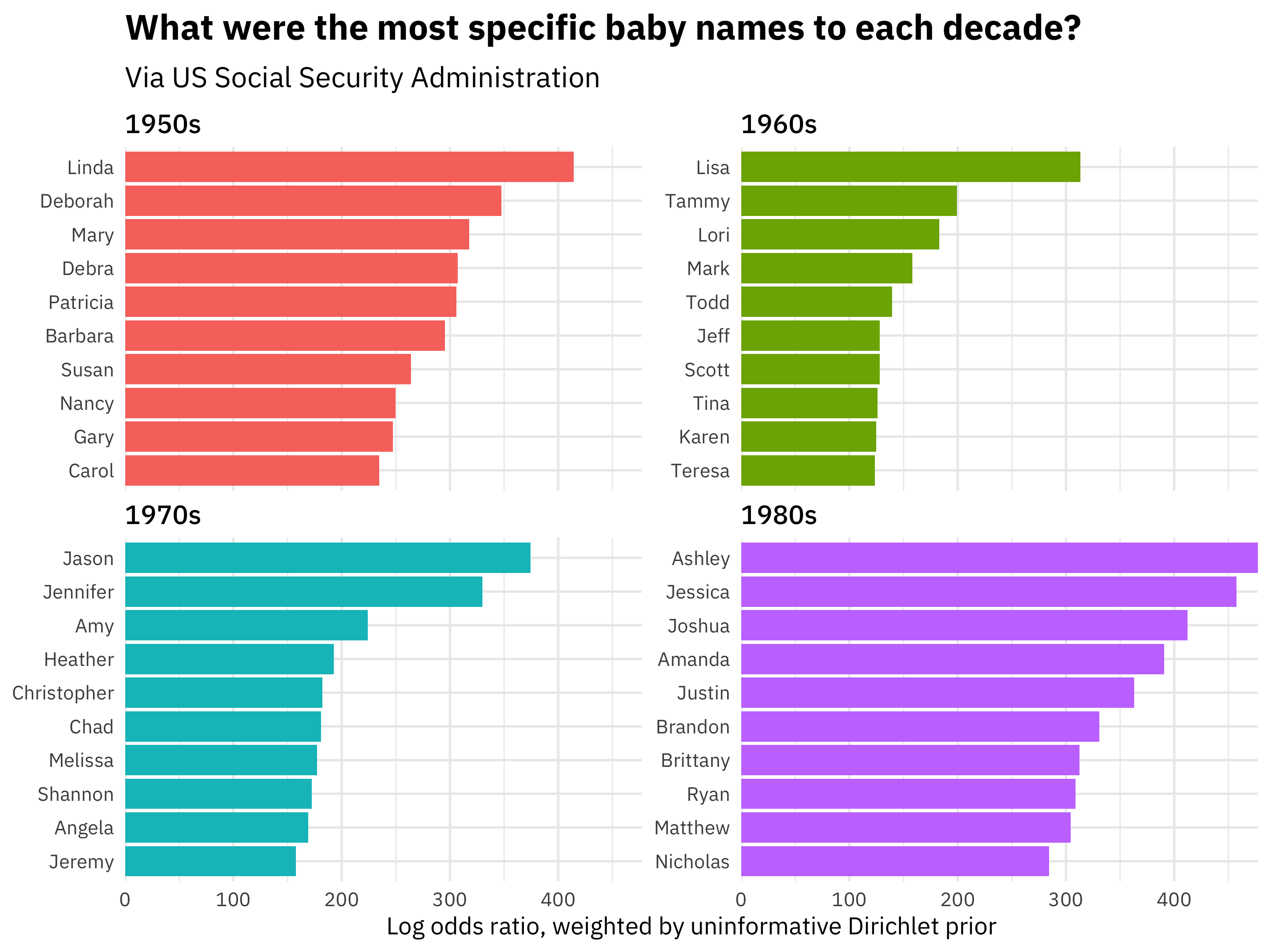



Introducing Tidylo R Bloggers




Log Odds Ratio Plot Of Tnt Vs Diagnoses Download Scientific Diagram



Plos One Physical Activity Sedentary Behavior Cardiorespiratory Fitness And Metabolic Syndrome In Adolescents Systematic Review And Meta Analysis Of Observational Evidence
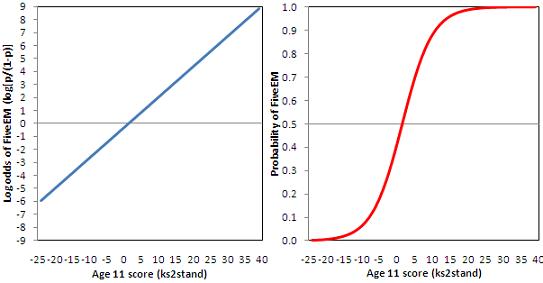



4 5 Interpreting Logistic Equations




The Log Odds Ratios Of The Depths At Heterozygous Snp Positions In The Download Scientific Diagram




What And Why Of Log Odds What Are Log Odds And Why Are They By Piyush Agarwal Towards Data Science




Volcano Plot Depicting Log Odds Ratios And P Values Of The Download Scientific Diagram
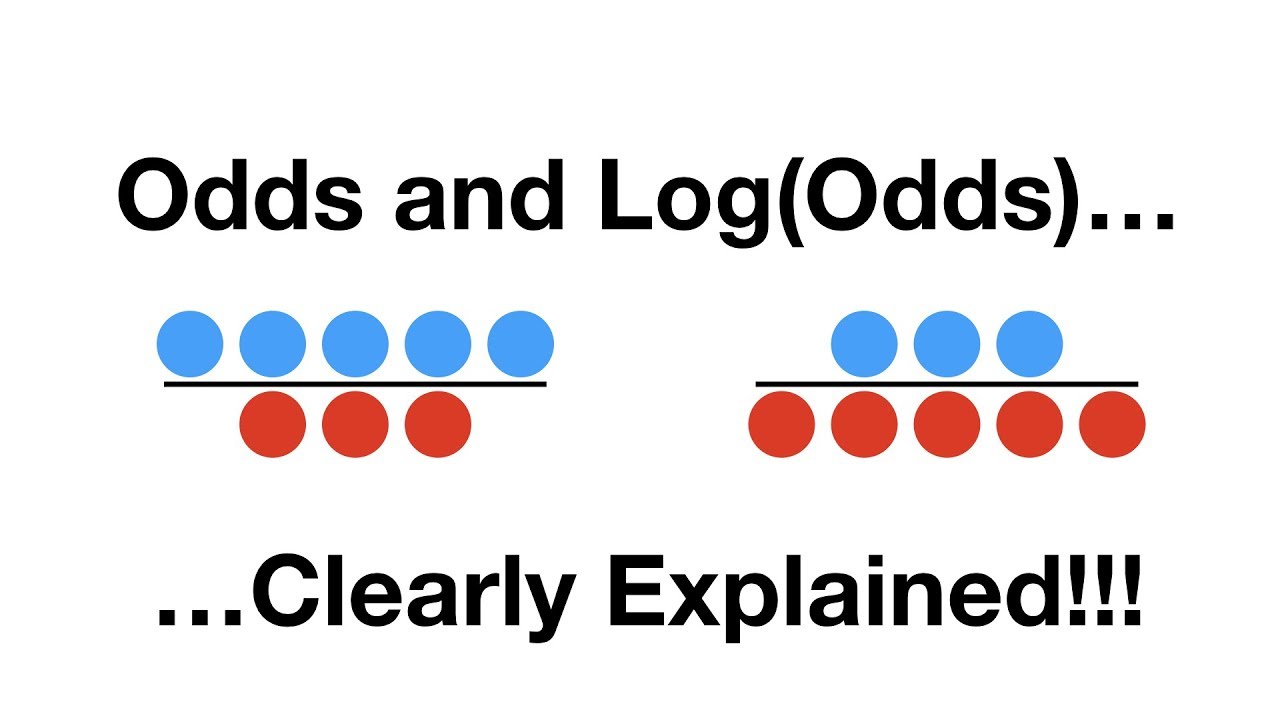



Odds And Log Odds Clearly Explained Youtube




Relative Bias Against Log Odds Ratio For The Chapman And Chao Estimators Download Scientific Diagram
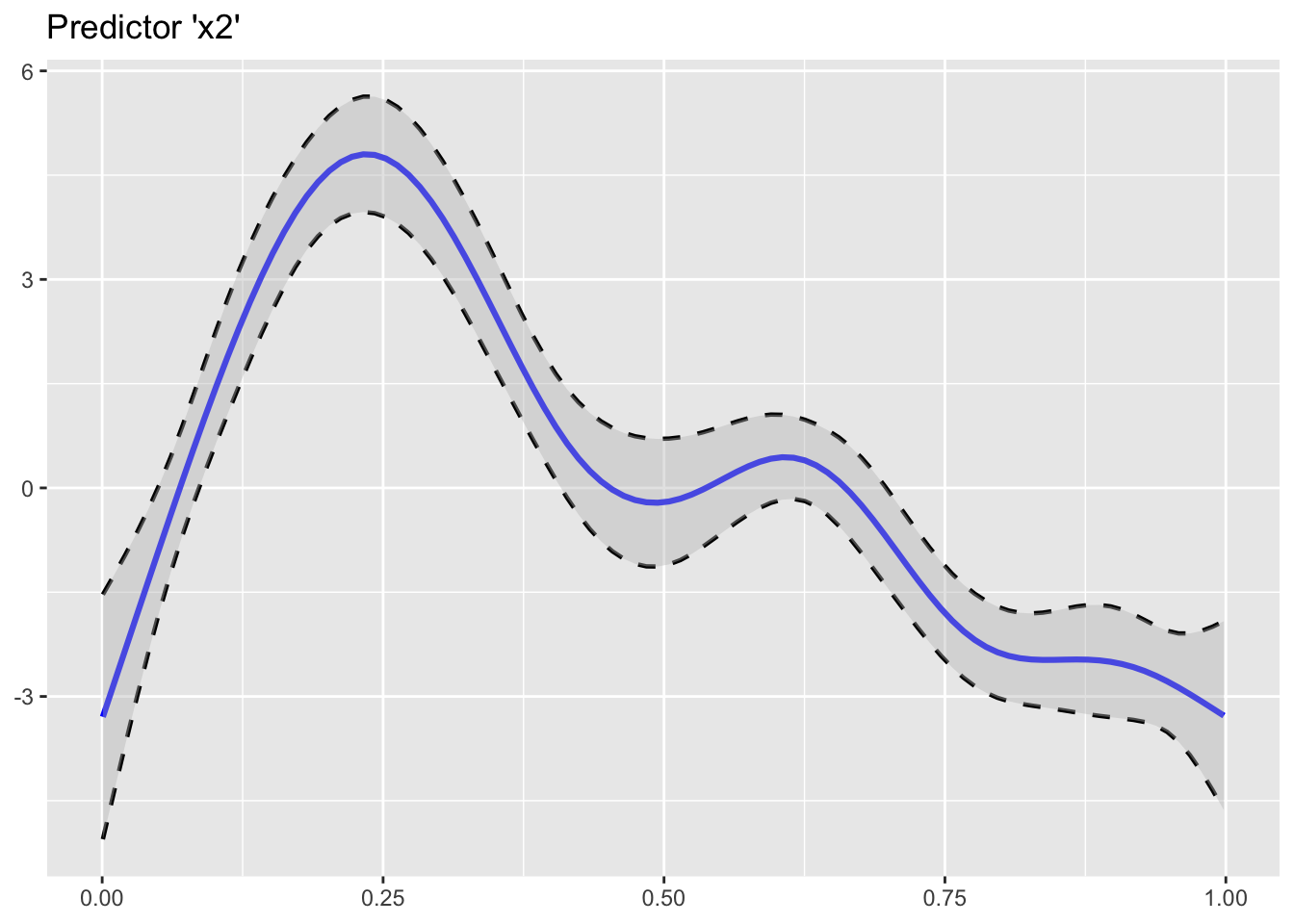



Introducing R Package Oddsratio Patrick Schratz
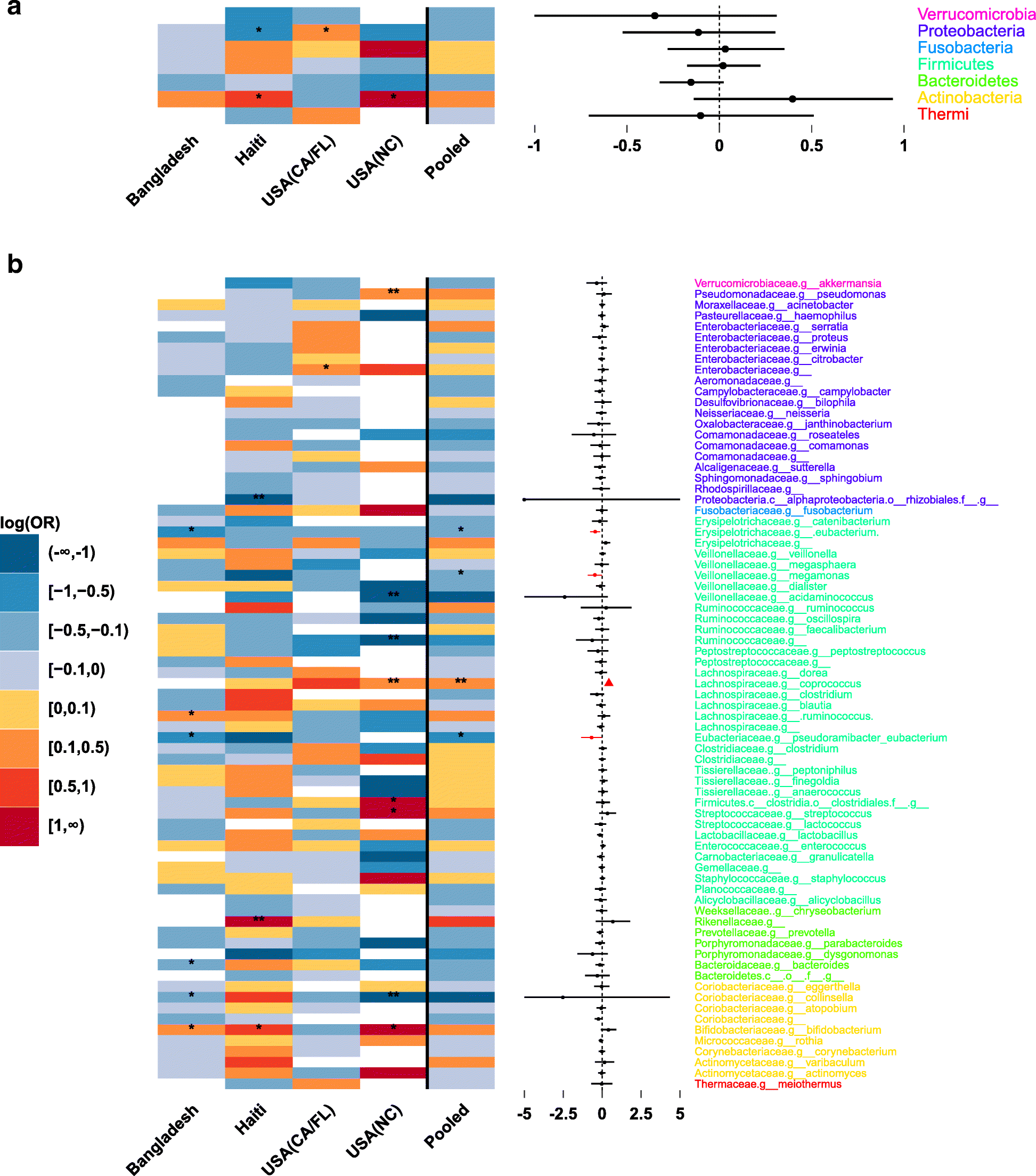



Metamicrobiomer An R Package For Analysis Of Microbiome Relative Abundance Data Using Zero Inflated Beta Gamlss And Meta Analysis Across Studies Using Random Effects Models Bmc Bioinformatics Full Text
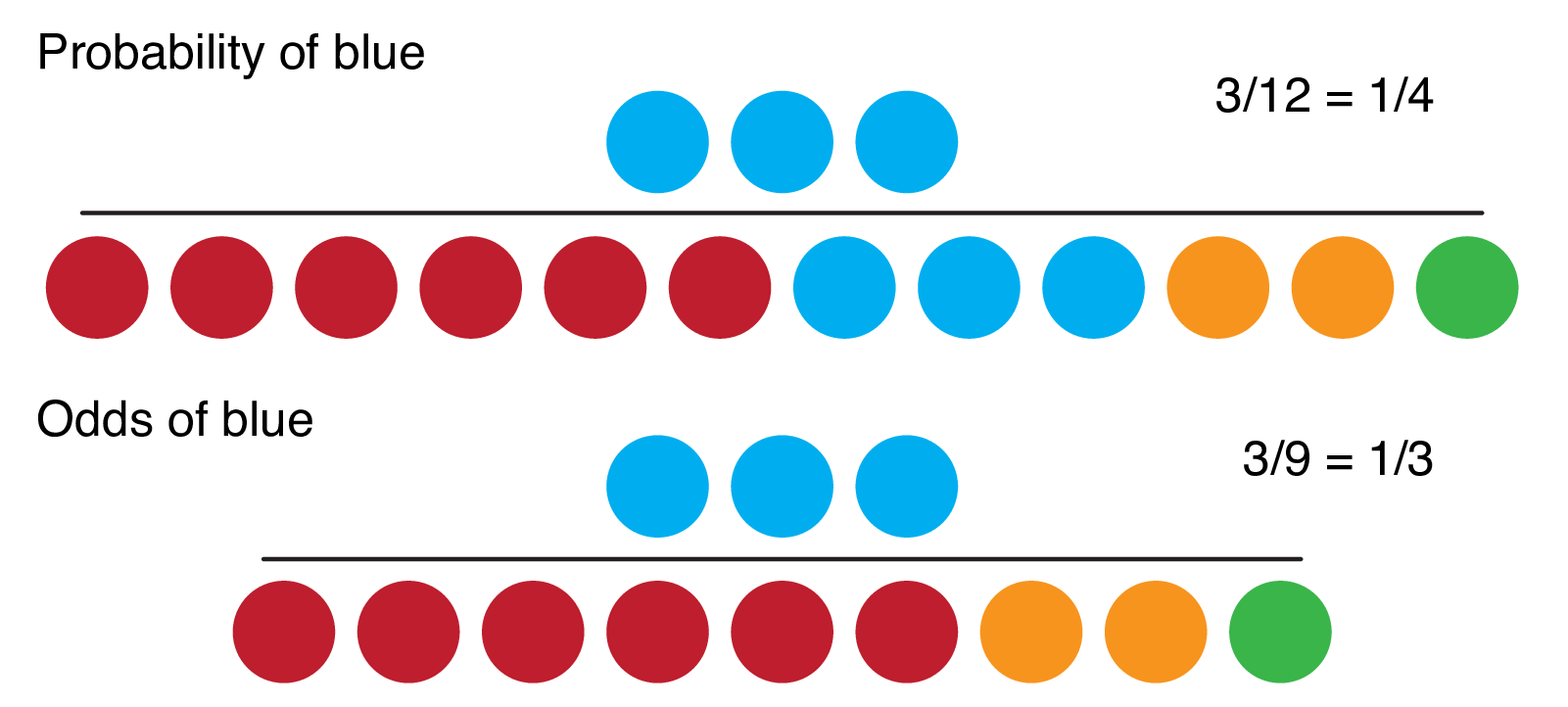



9 2 Binary Logistic Regression R For Health Data Science



Much Ado About Nothing Logit Vs Probit Stata Output Log Odds Vs Odds Ratio




Distribution Of The Log Odds Ratio S In Folded Unfolded And Download Scientific Diagram




Diagnostic Odds Ratio Wikipedia
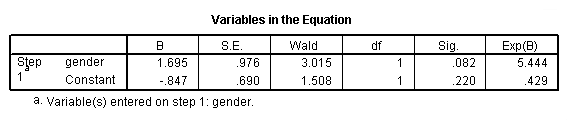



How Do I Interpret Odds Ratios In Logistic Regression Spss Faq




Log Odds Ratios Estimates And P Values For Contrasts Download Table




Odds Ratio Wikipedia
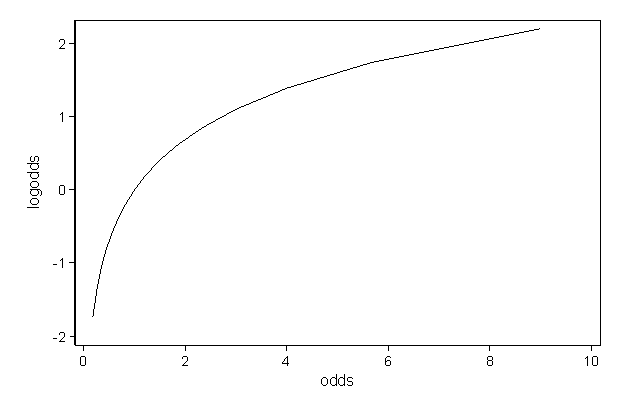



Faq How Do I Interpret Odds Ratios In Logistic Regression




2 Regression Results Logit Specification Log Odds Ratio Download Table



Odds Ratios Need To Be Graphed On Log Scales Andrew Wheeler



2



Relative Risk Ratios And Odds Ratios




Why Do So Many Practicing Data Scientists Not Understand Logistic Regression R Y X R




Strategies For Graphing Distributions Of Log Odds Estimates And The Corresponding Odds Ratios Modeling The Stan Forums




Plotting Odds Ratio Vs Continuous Variable In Stata Stack Overflow
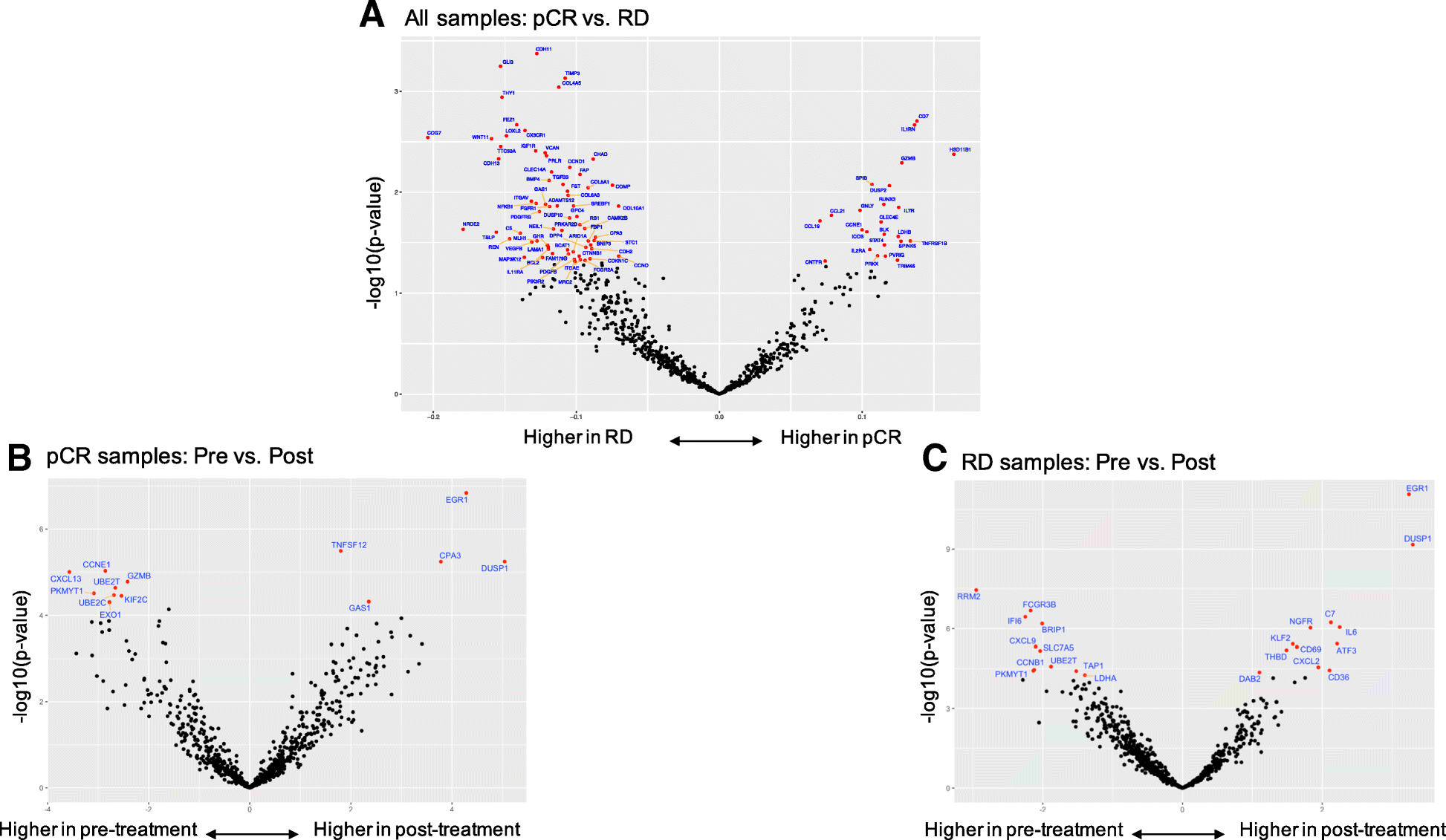



Immune Profiling Of Pre And Post Treatment Breast Cancer Tissues From The Swog S0800 Neoadjuvant Trial Journal For Immunotherapy Of Cancer Full Text
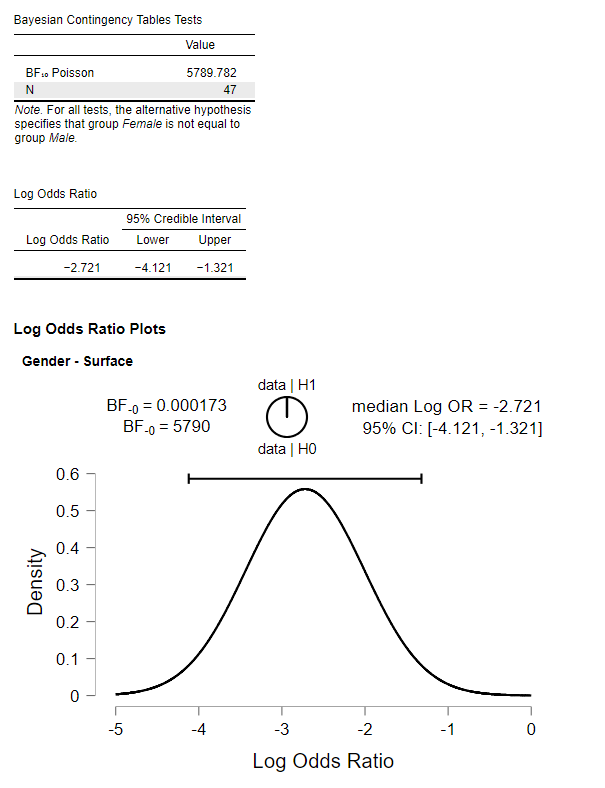



Log Odds Ratio Plots Not Correct Issue 595 Jasp Stats Jasp Issues Github




Statistics Sunday Everyone Loves A Log Odds Ratio Deeply Trivial




Funnel Plots For Detecting Bias In Meta Analysis Journal Of Clinical Epidemiology
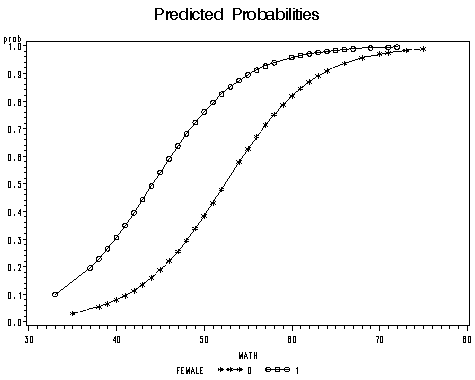



Proc Logistic And Logistic Regression Models
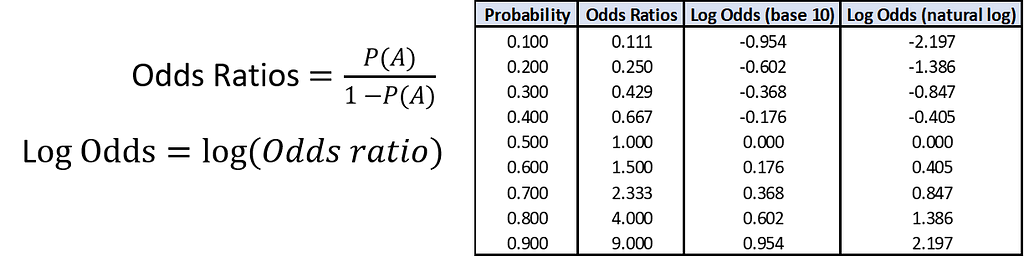



Into The Logistic Regression Towards Ai The World S Leading Ai And Technology Publication
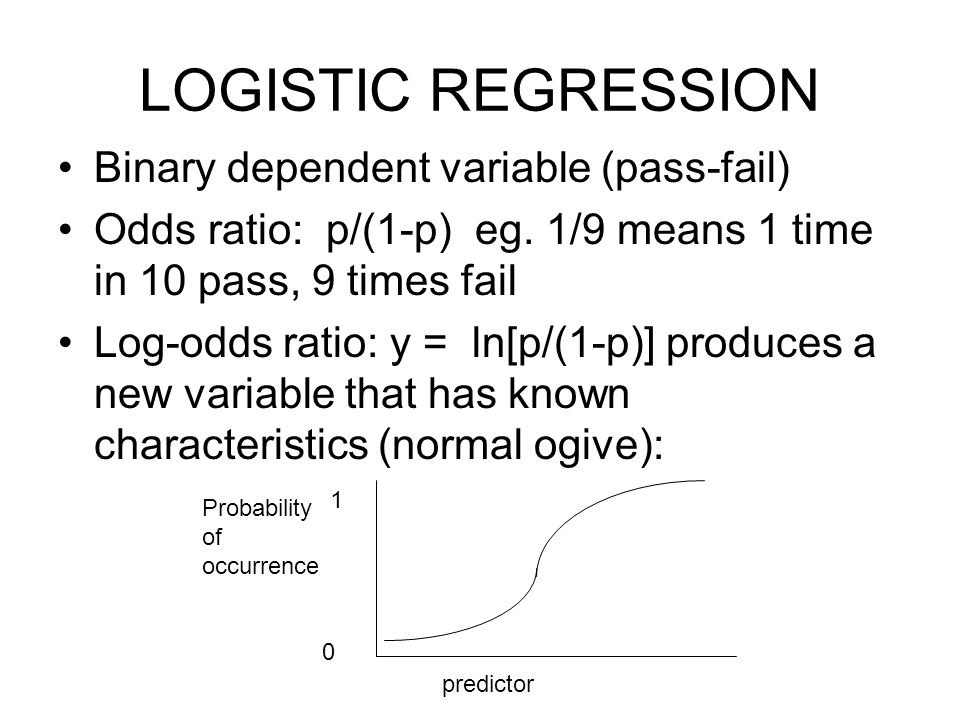



Logistic Regression Binary Dependent Variable Pass Fail Odds Ratio P 1 P Eg 1 9 Means 1 Time In 10 Pass 9 Times Fail Log Odds Ratio Y Ln P 1 P Ppt Download
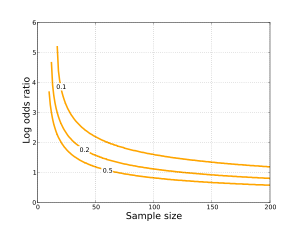



Odds Ratio Wikipedia
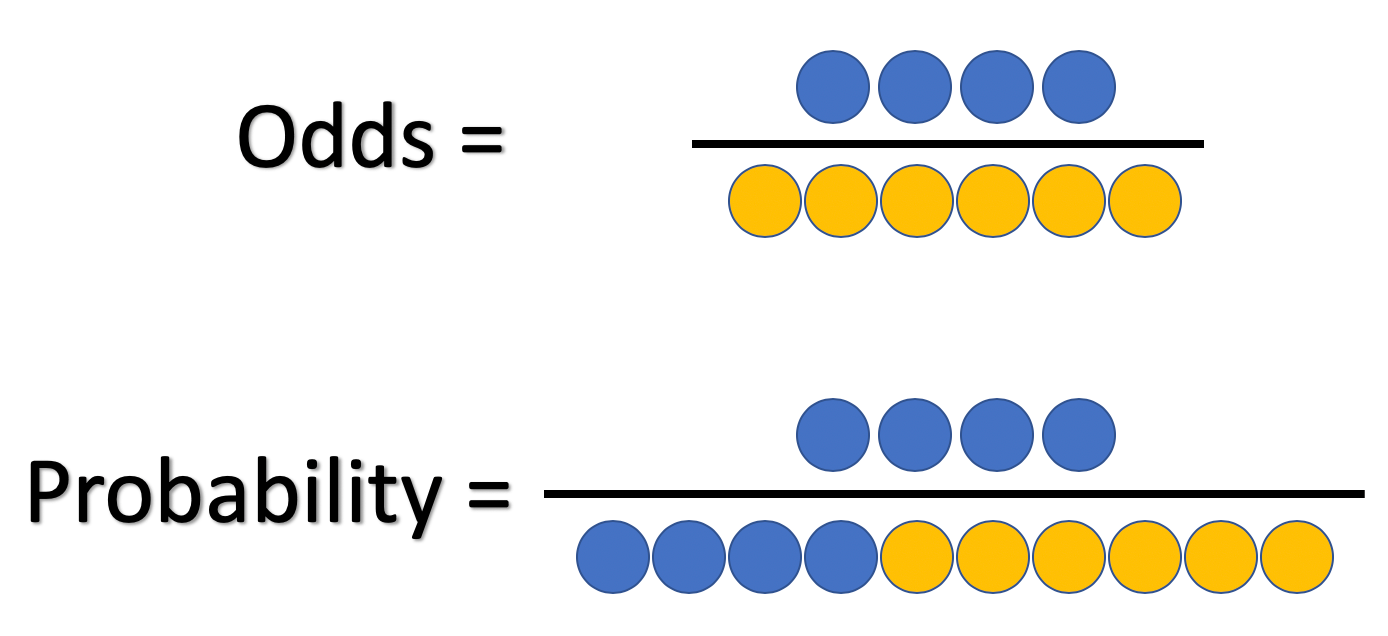



What And Why Of Log Odds What Are Log Odds And Why Are They By Piyush Agarwal Towards Data Science




Meta Analysis Of Odds Ratios Current Good Practices Abstract Europe Pmc




Comparative Expression Profiling Log Fold Change M Vs Log Odds Download Scientific Diagram
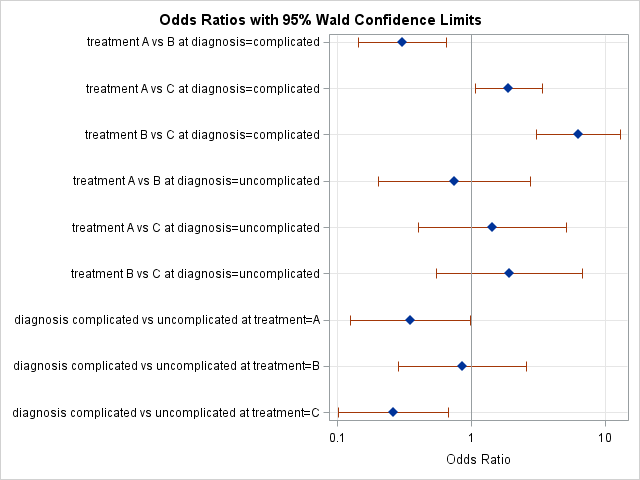



Odds Ratio Plots With A Logarithmic Scale In Sas The Do Loop




Funnel Plot Of Standard Error By Log Odds Ratio For Assessing Download Scientific Diagram